by Harley Beattie, Knowledge Transfer Partnership Associate.
1. Introduction
"An engineering change is an alteration made to parts, drawings or software that have already been released during the product design process. The change can be of any size or type; the change can involve any number of people and take any length of time." [1]
Changes become increasingly complex and expensive the later they are made in the development process. Therefore, an optimised ECM process is crucial for a successful development cycle. Fricke[2] defined five different ECM strategies;
- ‘Less’ - reducing the number of ECs.
- ‘Earlier’ (also referred to as front-loading) - early detection and implementation of changes to minimise the cost of its implementation.
- ‘Effective’ - accurate assessment of ECs to ensure that they are necessary and beneficial.
- ‘Efficient’ aims at the implementation of ECs by making the best use of resources.
- ‘Better’ - reviewing and evaluating ECs post implementation to document lessons learned and best practise. [3]
As the Covid-19 pandemic has illustrated, the automotive industry is becoming increasingly volatile and complex, with this trend set to continue as manufacturers strive to meet zero tailpipe emission targets. This uncertainty coupled with the rapid technological developments expected in the next 10 years with alternative powertrains and autonomous control systems means there will be increasing need for state-of-the-art ECM.
Many current ECM systems are designed for robust approval in a slower paced market, falling into the ‘Less’, ‘Earlier’ and ‘Effective’ strategies. As traditional powertrains begin to disappear, these workflows and systems will need to adapt to meet the demands of a now highly innovative and dynamic industry, pushing towards ‘Efficient’, ‘Better’, and a new Agile ECM strategy. This report outlines some of the industry changes likely to occur within the next 10 years, and how ECM must evolve to fulfil the new and changing demands of the automotive industry.
2. Green mobility
2.1. Zero tailpipe emissions by 2030
Legislation aimed at reducing tailpipe emissions is the main driving force behind the shift from traditional fossil fuels to electric and alternatively fuelled vehicles. Both the UK and EU have set an ambitious target of phasing out the sale of all tailpipe-emitting cars and vans by 2035 [4, 5].
The UK’s plan consists of 2 key steps:
- Phase-out date for the sale of new petrol and diesel cars and vans brought forward to 2030. Hybrid vehicles do not fall under this category.
- All new cars and vans be fully zero emission at the tailpipe from 2035. [5, 6]
This will force manufacturers to rapidly develop electric powertrains to meet this deadline. In 2020 29% of car sales in the UK has electrified powertrains, however, only 7% of sales were BEV (Figure 1) [7]. This is encouraging for Step 1 of the UK government’s plan to reduce tailpipe emissions, which currently appears to be achievable by 2030. However, with only an additional 5 years to convert 93% of current sales to fully electric (or alternative fuels such as hydrogen), the automotive sector will experience a rapid shift to this market. A PWC report predicts that globally, over 55% of all new car sales will be BEV by 2030 and over 95% of new car sales are expected to be partially electrified by 2030 [8]. This is reflected in Figure 2, which indicated a predicted increase from 8.5 to 116 million BEVs globally by 2030.
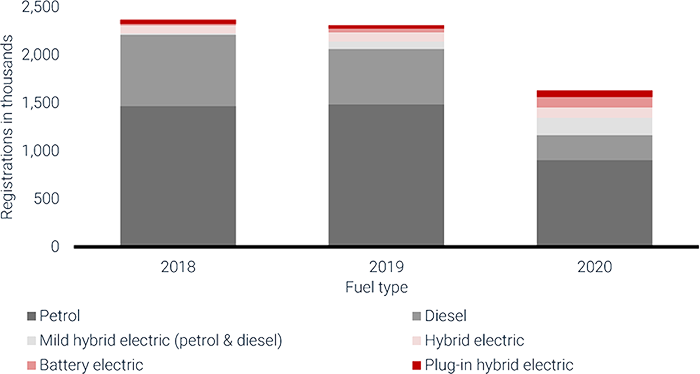


Figure 1: UK Car sales by fuel type 2018-2020 [7]
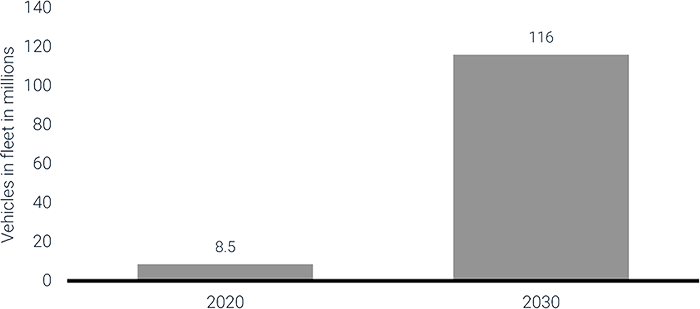

Figure 2: Projected size of the global electric vehicle fleet between 2020 and 2030 (in million units)[9]
The increasingly rapid shift to battery technology has potential to put strain on an already struggling supply chain. The Covid-19 pandemic highlighted risks in the semiconductor supply chain [10], and there are worsening bottlenecks in the supply of lithium and other raw materials required for battery manufacture [11-13]. This is driving significant investment in battery R&D, as large OEMs aim to gain more control of the battery supply chain via vertical integration of the production process [11]. Tesla is currently leading the charge, with European OEM’s such as VW aiming to catch up having recently announced major investment into battery manufacture [14, 15].
ECM
With rapid development in the EV space expected in the next 5-10 years, ECM will have to adapt to facilitating new vehicle architecture. Several EV start-ups have proved successful in this, having had the opportunity to design their ECM from scratch. However, large OEMs may struggle to adapt existing processes and tools to significantly different vehicle types. Furthermore, existing vehicle manufacturers will continue to produce petrol/diesel, PHEV and EV lines in parallel during the transition phase over the next 10 years. OEMs must choose whether to adapt current systems to work with all vehicle types, or whether to invest in designing and implementing new ECM processes. This provides an opportunity to create more agile, future proof ECM processes which can process increased software integration and IoT devices within vehicle designs more efficiently.
Another ECM consideration will be the volatile and rapidly changing supply chain. Rapidly emerging EV technologies, such as Tesla’s 4680 ‘tab-less’ cell design [16], coupled with potential global shortages of EV powertrain components may result in more changes from engineering and procurement throughout the lifecycle of the vehicle. This will require an agile ECM system that can facilitate rapid change whilst accurately estimating impact.
2.2. Paris Agreement and carbon reporting
In addition to legislation change driving the rapid development of electric vehicles, green policies such as the Streamlined Energy and Carbon Reporting (SECR) [17] is also forcing automotive companies to reflect and act on their own carbon output. As of April 2019, large companies in the UK are required to report energy use and carbon emissions as part of their annual reporting.
Carbon reporting such as this will continue to increase globally, in an effort to enforce Article Six of the Paris Agreement [18].
ECM
This will require a new approach to data collection and analysis, including ECM. Previously the impact of a change was primarily quantified by cost, weight, and the resulting propagated changes within a design. With an increased focus on transparency of carbon footprint and waste, ECM professionals may be required to calculate and report the environmental impact of a new material choice e.g. impact of scrapping obsolete stock, vehicle efficiency impact and so forth. This additional consideration during the design change process will result in increased complexity in ECM. Investment will be required in ECM to create workflows and tools to aid increasingly complex impact prediction.
3. Agile in Automotive
Software in modern vehicles is rapidly evolving due to innovation in EV and autonomous technology. With the phase out of traditional powertrains in the next 5-10 years, the automotive industry will see a significant shift towards becoming software centric. In addition to dealing with increasingly complex software, the race to capture the EV market has put pressure on companies to shorten time to market. In the current market, it is a competitive advantage to develop and innovative software at high pace, as Tesla have illustrated [19].
As a result, established plan-driven or waterfall development processes cannot serve this sector effectively [20]. Agile development has been established in the software industry for over 20 years, the benefits of which are well known. By taking a iterative and incremental approach, product development is more customer orientated and able to react to changing market conditions [21]. Adopting agile development strategies are becoming a popular method for tackling the rapidly evolving automotive market, as several EV Start-ups have demonstrated. For example, Arrival employs agile techniques in its operational structure, distributing in-house manufacturer of many components between ‘micro factories’. These deployable micro-factories are designed to produce any vehicle line on demand, allowing arrival to react quickly to changing markets and significantly enhancing their competitive edge [22]. The adoption of agile techniques is clearly advantageous for start-ups who can design manufacturing and workflow processes from scratch, but large OEMs may struggle to replicate this. There are also challenges with adopting agile in automotive, such as accommodating parallel development of hardware and software, and rigorous testing and approval for safety critical items. This may lead to an automotive specific definition of agile development as it becomes adopted more widely by OEMs.
ECM
By facilitating increased customer orientation and adaptability to a rapidly changing market, agile processes will increase the workload of ECM. To process a greater number of changes in a reduced time frame, an efficient ECM process will be required. Impact analysis software could be used to empower engineers to make changes with minimal senior stakeholder engagement. Leaner workflows designed for each stage of development and risk can also help expedite the implementation of engineering changes with minimal resource.
Implementing such a system in an established OEM may come with its own additional challenges, including integrating with legacy software, robust traditional manufacturing processes and lack of buy in from senior stakeholders.
4. Technology driving change
4.1. Autonomous vehicles
As with electric vehicles, sales of autonomous vehicles are expected to increase rapidly in the next 10 years as the supporting technology advances and gains trust from the consumer market. Figure 3 shows the forecasted increase in global sales of autonomous vehicles (all degrees of automation.)
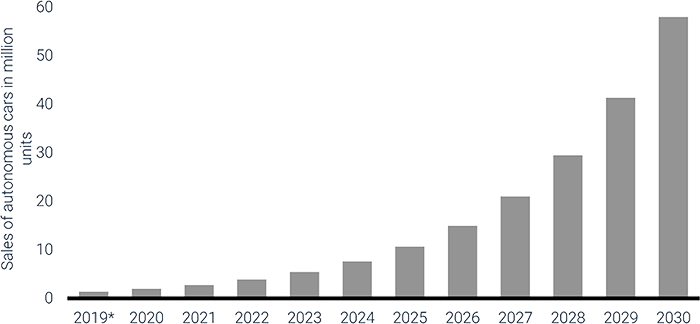

Figure 3: Projected sales of autonomous vehicles worldwide from 2019 to 2030 (in million units). *Estimated rather than projected [23]
As the autonomous vehicle market grows, it is expected that the adoption of ‘smart’ technologies will increase rapidly, introducing novel concepts such as connected, self-aware vehicle fleets, shared mobility on demand and internet-connected ECUs providing live data and vehicle insights [24].
ECM
One of the key ECM challenges will be issuing software updates in the field. Although software updates like this are not new in the computing sector, it is largely new to vehicle manufacture. Many established OEMs do not have change management systems designed for this functionality. Additional complexity arises when software is used across multiple vehicle lines, where integrated software and hardware must be linked. A block change system may be a solution in this case, at the cost of agility in the process.
With autonomous vehicles software will play a critical role in passenger safety, and the ECM processes in place must reflect this. Therefore, ECM will need to strike a balance between agility and rigour to ensure the safety of the final product.
4.2. Novel EV technologies
As with many modern industries, the automotive industry may see a shift towards subscription-based models as opposed to one-time purchase. Technological advances in battery design and IOT connectivity has facilitated the emergence of novel technologies such as Battery as a Service (BaaS). One of the main consumer concerns surrounding BEV’s is the cost, maintenance, and lifespan of the vehicle battery. By offering BaaS, manufacturers hope to eliminate these barriers to widespread adoption.
Last year, NIO, a Chinese based automotive manufacturer, launched its BaaS service with subscriptions for a 70- kWh battery pack, for £110 per month. By opting for the battery ‘subscription’, buyers were offered a £7,250 (~$10,000) discount on vehicle purchase. NIO have also invested in battery swapping infrastructure, so customers can replace discharged batteries, eliminating charge waiting time [25].
ECM
Committing to large scale infrastructure and long-term subscription services places greater pressure on ECM to minimise or eliminate postproduction changes that could affect the battery or any ancillary components. Initial change management for design must introduce a new degree of rigour and details analysis to ensure this, and incorporate Design for Change (DfC) at an early stage to future proof the design and supporting infrastructure.
When designing new vehicles, changes must be thoroughly checked to ensure they will not affect swap stations or the interfacing battery design. This may be more akin to procedures adopted in aerospace projects: reduced agility, increased scale and complexity, and a rigorous approval process.
If new battery architecture is required, ECM must be able calculate the associated impacts, and facilitate this large-scale block change. This includes the impacts on both the vehicle design and the battery-swap network, introducing a new area of expertise that automotive ECM professionals must acquire.
4.3. AR/VR
Augmented reality (AR) and virtual reality (VR) are relatively well-established technologies which are beginning to find their place in the engineering sector, for example as an immersive aid to CAD design [26], virtual validation and collaborative design [27]. As this technology becomes more accessible, its use in the engineering space will expand. One of the less obvious applications of this technology may be in ECM.
One of the key drivers of engineering change is customer driven requirements change. This is more prevalent in bespoke, niche or luxury vehicle markets, as the customers satisfaction is often a top priority. In extreme cases, customers may not finalise requirements until a physical mock-up can be produced, which is time consuming, expensive, and inefficient. By using AR/VR technology, the need for a physical mock-up could be mitigated, helping customers finalise requirements much earlier in the development phase, and at reduced cost. This in turn has the potential to significantly reduce the number of late engineering changes.
The same principle applies to the design process. Engineering VR technology provider Varjo claim that designers can spot more than 80% of the problems that usually go unnoticed when experiencing and modifying projects on a 1:1 scale in an immersive environment [27]. This, coupled with enhanced collaborative engineering via VR could further reduce the number of design iterations, optimise solutions faster and hence reduce the number of ECNs raised throughout the development cycle.
4.4. AI and Machine Learning
In addition to facilitating the development of autonomous vehicles, Artificial Intelligence (AI) and Machine Learning have the potential to significantly improve ECM processes and systems. When an ECN is raised, several impacts must be estimated including cost, weight, supplier impact, change propagation and (potentially) environmental impact. In many cases this is estimated manually by the engineer, with more advanced systems using Function–behaviour–structure (FBS) linkage ontology [28], model-based systems [29] and various other Change Prediction Methods (CPM)[30] to reference similar changes to aid impact assessment. Applying machine learning to a database of ECNs and resulting impacts could improve impact assessment accuracy, as estimates will not vary with the experience of the engineer. Such technology may also have the ability to help predict potential changes by considering incoming legislation changes, failed simulations, or supply chain issues [31]. This approach has already been investigated in the aerospace industry- a case study from [32] indicated the operational complexity of a service project could be predicted with a 70% accuracy, using only 30% of the project data using a machine learning model.
5. Summary of ECM challenges in Automotive
5.1. Green mobility
- Rapid technology development > significant increase in revisions of released designs to expedite development.
- Pressure on supply chain for EV (raw materials for current battery technology, semiconductor chips etc) > strain on procurement and increased ECs.
- Legislation on carbon/waste reporting > additional impact to consider during EC process.
5.2. Technology driving change
- The use of AR & VR to help freeze customer requirements earlier and without expensive mock-ups > reduce number of EC’s during product development.
- Claims that AR & VR can optimise solutions easier and enable engineers to spot 80% of previously missed errors > reduced strain on ECM.
- Using AI to learn from previous ECN’s > more accurate impact and risk assessments.
5.3. Agile in Automotive
- Increasingly software centric > ECM processes will adapt to implement integrated software & hardware changes.
- Agile development strategies > ECM will process greater number of changes quicker (lean/agile workflows), whilst ensuring robust approval for safety critical elements.
- ECM will have to facilitate postproduction, block changes of software in the field.
6. ECM in other sectors
Many of the driving factors behind the changes in automotive are also generating innovation in other spaces. The shift in global consciousness regarding energy sustainability coupled with increasingly accessible innovative technology will have a much wider affect than simply the automotive industry. ECM needs can vary quite significantly between sectors. QR has benchmarked some of these sectors including aerospace and defence (maritime) against the automotive industry, shown in Figure 4.
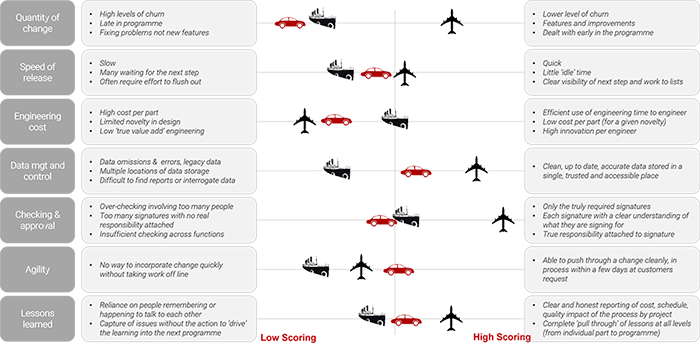

Figure 4: ECM Benchmarking against automotive.
6.1. Energy
The Energy sector is expected to “see an unprecedented level of change” both in the immediate future and the coming decade [33]. With innovative consumer items such as electric vehicles rising in popularity, and the closure of all coal power plants by 2025, the energy sector faces the challenge of decarbonising and decentralising whilst maintaining a secure power supply [33].
The shift to decarbonise is a global effort, with legislation such as the Paris Agreement driving change. In this case we shall focus on the UK as an example.
The UK government has outlined three main goals for energy supply: decarbonisation, security, and affordability. A report by Arup [34] has highlighted that many solutions will be required to achieve this, and flexibility in system architecture, operation and regulatory frameworks is essential. In terms of practical steps, the Arup report predicts that the installation of solar and wind will need to triple, with ‘micro grids’ located at universities or industrial parks potentially providing 34-45% of the nations generation capacity. This will create a boom in the development, production and distribution of green energy generators such as solar panels, wind turbines and other innovative technologies.
ECM
The boom in the green energy sector coupled with rapid innovation (like that seen in the automotive space) will result in the need for more effective engineering data management including ECM. As new businesses emerge and established engineering companies diversify, there will be a need for expert consultation regarding the design and implementation of ECM systems.
The manufacture of solar panels and wind turbine share some similarities with vehicles, with production volumes and development lifecycle lengths are of similar magnitude. Although solar panels and turbines may have fewer components than a vehicle, the manufacturing process of components is significantly more complex. Despite this, the knowledge from established ECM systems in the Automotive industry could be used to aid the design of critical features such as impact assessment and workflow design with appropriate stakeholder management.
6.2. Aerospace
As shown on Figure 4, ECM in aerospace varies quite significantly from the automotive sector. Aerospace is a heavily regulated, safety critical industry, in which the size of operations and development cycle timescale is a magnitude larger than automotive, hence rigour and meticulous planning is favoured over agility and innovation. The lifespan of a commercial aircraft can be over 30 years, hence, the industry is slow moving compared to automotive. The Airbus A320 design has been in production since 1986, with only incremental improvements over this 35-year period.
Despite this, a 2019 report from Airbus predicts that within the next 20 years, a growing global middle class and increased demand for airfreight will create demand for 39,210 new aircraft. This includes replacing over 14,200 aircraft currently in service, in addition to doubling the global fleet [35].
The climate change crisis will also force the commercial aerospace sector to change radically in the coming decades. Whilst this is a technical challenge, serval innovations are emerging in this sector. This year Rolls-Royce tested its electric powered prototype ‘Sprit of innovation’. Rolls-Royce claim the advanced battery and propulsion technology developed will help the industry take a step closer to zero emissions [36].
ECM
A new wave of innovation in the commercial aerospace sector will generate demand for smarter, more efficient ECM. In addition to rigorous assessment and approval processes, this industry would benefit significantly from accurate concern ageing and cost prediction. Machine learning models could be used to achieve this, enabling better time-to-market planning and budget control. Machine learning techniques have been proved successful in predicting characteristics for service projects in aerospace [32], as mentioned in Section 4.4. Applying this technology to ECM could help predict concern ageing, cost of change and impact to the product development lifecycle.
References
- Kattner, N., T. Wang, and U. Lindemann, Performance metrics in engineering change management - Key Performance Indicators and engineering change performance levels. 2016, IEEE. p. 1180-1184.
- Fricke, E., et al., Coping with changes: Causes, findings, and strategies. Systems engineering, 2000. 3(4): p. 169-179.
- Iakymenko, N., et al., Status of engineering change management in the engineer-to-order production environment: insights from a multiple case study. International Journal of Production Research, 2020. 58(15): p. 4506-4528.
- Bannon, E. EU climate plan will make emissions-free cars accessible for all. 2021 [cited 2021 31/08/2021].
- Transport, D.F., Decarbonising Transport: A Better, Greener Britain, D.F. Transport, Editor. 2021.
- Transport, D.f. Government takes historic step towards net-zero with end of sale of new petrol and diesel cars by 2030. 2020.
- SMMT. UK automotive looks to green recovery strategy after -29.4% fall in new car registrations in 2020. 2021.
- PWC, Five trends transforming the Automotive Industry. 2018.
- Colin McKerracher, e.a., Electric Vehicle Outlook 2020. 2020: BloombergNEF.
- Baraniuk, C. Why is there a chip shortage? 2021 [cited 02/09/21].
- Harrison, D., Electric Vehicle Battery Supply Chain Analysis: How Battery Demand and Production are Reshaping the Automotive Indusry. 2021, ABB: Automotive from Ultima Media.
- Lee, A., Tight Battery Market Is Next Test for EVs After Chip Crisis. Bloomberg.com, 2021: p. 1-2.
- Winton, N., Battery Scarcity Could Dwarf Chip Shortage Impact On Global Auto Sales, in Forbes. 2021.
- Hetzner, C., VW takes a page from Tesla on batteries: Move to manufacture aims for EV control. Automotive News, 2021. 95(6978): p. 3-4.
- Volkwagen, Volkswagen Group starts battery cell development and production in Salzgitter, in Media Information. 2019: Salzgitter.
- Ulrich, L. Is Elon Musk Back In “Production Hell” With Tesla’s 4680 Battery? 2021 [cited 02/09/21].
- Environmental Reporting Guidelines: Including streamlined energy and carbon reporting guidance, F.R.A.a.D.f.B. Department for Environment, Energy & Industrial Strategy, Editor. 2019, HM Government.
- Nations, U., Paris Agreement. 2015.
- Hohl, P., et al., Forces that Prevent Agile Adoption in the Automotive Domain. 2016, Cham: Springer International Publishing: Cham. p. 468-476.
- Katumba, B. and E. Knauss, Agile Development in Automotive Software Development: Challenges and Opportunities. 2014, Cham: Springer International Publishing: Cham. p. 33-47.
- Fritzsche, A., Implications of agile manufacturing in the automotive industry for order management in the factories-evidence from the practitioner’s perspective. Procedia CIRP, 2018. 72: p. 369-374.
- Arrival. The Arrival Microfactory. 2021.
- Markets, R.a., Autonomous Vehicle Market by Automation Level, by Application, by Component - Global Opportunity Analysis and Industry Forecast, 2020-2030. 2020: Statista.
- Autonomous Vehicle and Smart Traffic. 2020: IntechOpen.
- NIO. NIO Launches Battery as a Service. [cited 2020 01/08/21].
- Martin, P., et al., A VR-CAD Data Model for Immersive Design: The cRea-VR Proof of Concept. 2017, Cham: Springer International Publishing: Cham. p. 222-241.
- Varjo, Virtual and Mixed Reality for Industrial Design. 2021: Varjo HQ Vuorikatu Helsinki, Finland.
- Hamraz, B. and P.J. Clarkson, Industrial evaluation of FBS Linkage – a method to support engineering change management. Journal of Engineering Design, 2015. 26(1-3): p. 24-47.
- Meißner, M., et al., Model based systems engineering as enabler for rapid engineering change management. Procedia CIRP, 2021. 100: p. 61-66.
- Ariyo, O.O.E., C.M.; Clarkson, P.J., On The Use Of Functions, Behaviour And Structural Relations As Cues For Engineering Change Prediction, in DS 36: International Design Conference. 2006: Croatia. p. 773-782.
- English, T. How Machine Learning and AI Will Impact Engineering. 2020 [cited 26/08/21].
- Shi, L., et al., Learning to predict characteristics for engineering service projects. Artificial Intelligence for Engineering Design, Analysis and Manufacturing, 2016. 31(3): p. 313-326.
- UK, E., The Future of Energy. 2019.
- Arup, Energy systems: A view from 2035. What will a future energy market look like? 2019.
- Airbus, Global Market Forecast. Cities, Airports and Aircraft. 2019-2038. 2019: Blagnac Cedex, France.
- Rolls-Royce, Rolls-Royce’s all-electric ‘Spirit of Innovation’ takes to the skies for the first time, in Press Release. 2021.